How Big Tech Sees DeepSeek: Five Key Takeaways
On diffusion of innovation, the need for strong business models, lower inference costs benefiting apps and investing in infrastructure as a strategic advantage
This is a weekly newsletter about the business of the technology industry. To receive Tanay’s Newsletter in your inbox, subscribe here for free:
Hi friends,
Much has been said about DeepSeek, but I would argue that perspectives that matter most come from Big Tech executives since they’re the ones that are making the capital allocation and resource decisions around hundreds of billions of dollars and tens of thousands of researchers. This past week’s earnings calls provided a window into how these companies are digesting DeepSeek’s innovations, adapting their strategies, and positioning for what’s next.
Here are the five key takeaways on Deepseek from Amazon, Google, Microsoft, Meta, and Apple:
1. DeepSeek Represents Real Innovation in AI Training & Efficiency
Much has been made of whether Deepseek is a psyop from China and whether the $5.5M training number cited is real (It is not and only represents the potential cost of the final training run, as mentioned in the paper itself).
But Big Tech leaders broadly acknowledged DeepSeek’s novel training methods—especially its early application of reinforcement learning without human-in-the-loop fine-tuning—as a major innovation. In fact, Microsoft and Amazon have already made the Deepseek models available on their clouds.
Amazon’s Andy Jassy highlighted their novel techniques:
“Like many others, we were impressed with what DeepSeek has done. I think in part impressed with some of the training techniques, primarily in flipping the sequencing of reinforcement training—reinforcement learning being earlier and without the human-in-the-loop.”
Microsoft’s Satya Nadella noted the same:
“DeepSeek has had some real innovations. And that is some of the things that even OpenAI found in o-1."
Meta’s CEO Mark Zuckerberg acknowledged learning from them:
“There’s a number of novel things that they did that I think we’re still digesting. And there are a number of advances that we will hope to implement in our systems.”
Tim Cook praised their innovations in efficiency:
In general, I think innovation that drives efficiency is a good thing. And that's what you see in that model.
These comments reinforce that DeepSeek’s approach has pushed forward the efficiency of AI model development, particularly in reducing costs and increasing inference speed.
2. AI Model Leapfrogging & Commoditization Will Accelerate
A consistent theme was that DeepSeek’s advances are contributing to an environment of rapid model iteration and commoditization. Companies are quickly learning from each other, meaning that competitive advantages from model breakthroughs may not last long.
Amazon’s Jassy noted how competitive AI development has become:
“For those of us who are building frontier models, we're all working on the same types of things and we're all learning from one another. I think you have seen and will continue to see a lot of leapfrogging between us.”
Microsoft’s Nadella emphasized that improvements and techniques diffuse quickly:
“As soon as something novel emerges, it gets diffused through the entire industry. So, while DeepSeek is ahead in some areas today, these advantages won’t last long.”
“…obviously, now that all gets commoditized and it's going to get broadly used.”
Meta’s Zuckerberg confirmed that every major model release leads to industry-wide improvements:
“Every new company that has an advance… I expect the rest of the field to learn from it. That’s just how the industry moves forward.”
3. AI Compute Costs Will Fall—But Total AI Spend Will Grow as More Apps Emerge
A major theme across the earnings calls was that falling AI compute costs will increase overall AI spending rather than reduce it—a classic example of Jevons Paradox, where improvements in efficiency lead to greater overall consumption rather than decreased usage. Executives emphasized that as AI inference becomes cheaper, the real winners will be those building applications on top of models.
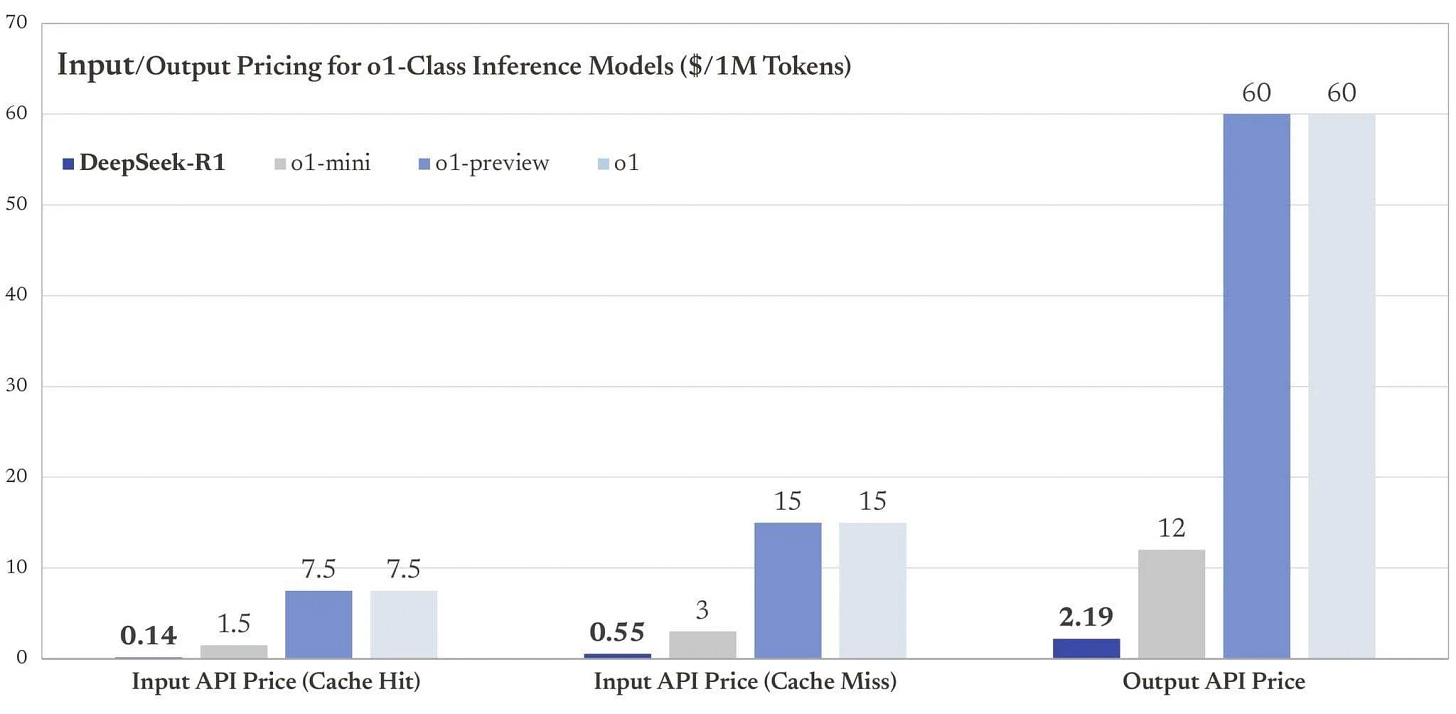
Amazon’s Jassy compared AI compute costs to the AWS cloud adoption curve:
“People assume that decreasing the cost of inference means less total AI spend. But we saw the same thing with cloud computing—once infrastructure costs fell, companies built even more applications. AI will follow the same pattern.”
Microsoft’s Nadella explicitly called out the application layer as the primary winner:
“The big beneficiaries of any software cycle like that is the customers, right? Because at the end of the day, if you think about it, right, what was the big lesson learned from client server to cloud? More people bought servers, except it was called cloud. And so when token prices fall, inference computing prices fall, that means people can consume more, and there will be more apps written.”
Meta’s Zuckerberg highlighted that higher-quality AI means more compute demand:
“One of the new properties that’s emerged is the ability to apply more compute at inference time in order to generate a higher level of intelligence and a higher quality of service.
The conclusion is that cheaper AI will lead to more AI, not less—increasing both enterprise adoption and total industry spend.
4. Inference Cost Shifts Favor Companies with Strong Business Models
Several executives noted that AI’s shift toward inference-heavy compute will reward companies with better monetization models.
Meta’s Zuckerberg framed it as a business model differentiation issue:
“As a company that has a strong business model to support this, I think that’s generally an advantage—that we’re now going to be able to provide a higher quality of service than others who don’t necessarily have the business model to support it on a sustainable basis.”
Google’s Pichai highlighted how AI will be monetized through cost-efficiency:
“AI efficiency will matter most in businesses with strong ROIC models. The reason we are so excited about the AI opportunity is, we know we can drive extraordinary use cases because the cost of actually using it is going to keep coming down.”
“The proportion of spend towards inference compared to training has been increasing, which is good because inference supports businesses with good ROIC.”
The implication: Companies that can monetize AI effectively—through subscriptions, enterprise integrations, or direct-to-consumer services—will be the ones to benefit most from AI’s cost curve improvements and shift towards inference time compute.
5. Investing in AI Infrastructure Remains a Strategic Advantage
Despite discussions around AI cost reductions, Big Tech remains aggressively investing in AI infrastructure, seeing it as a long-term strategic advantage. Executives from Amazon, Meta, Microsoft, and Google all emphasized continued high CapEx spend, reinforcing the belief that scale and access to compute will be a key differentiator in AI leadership.
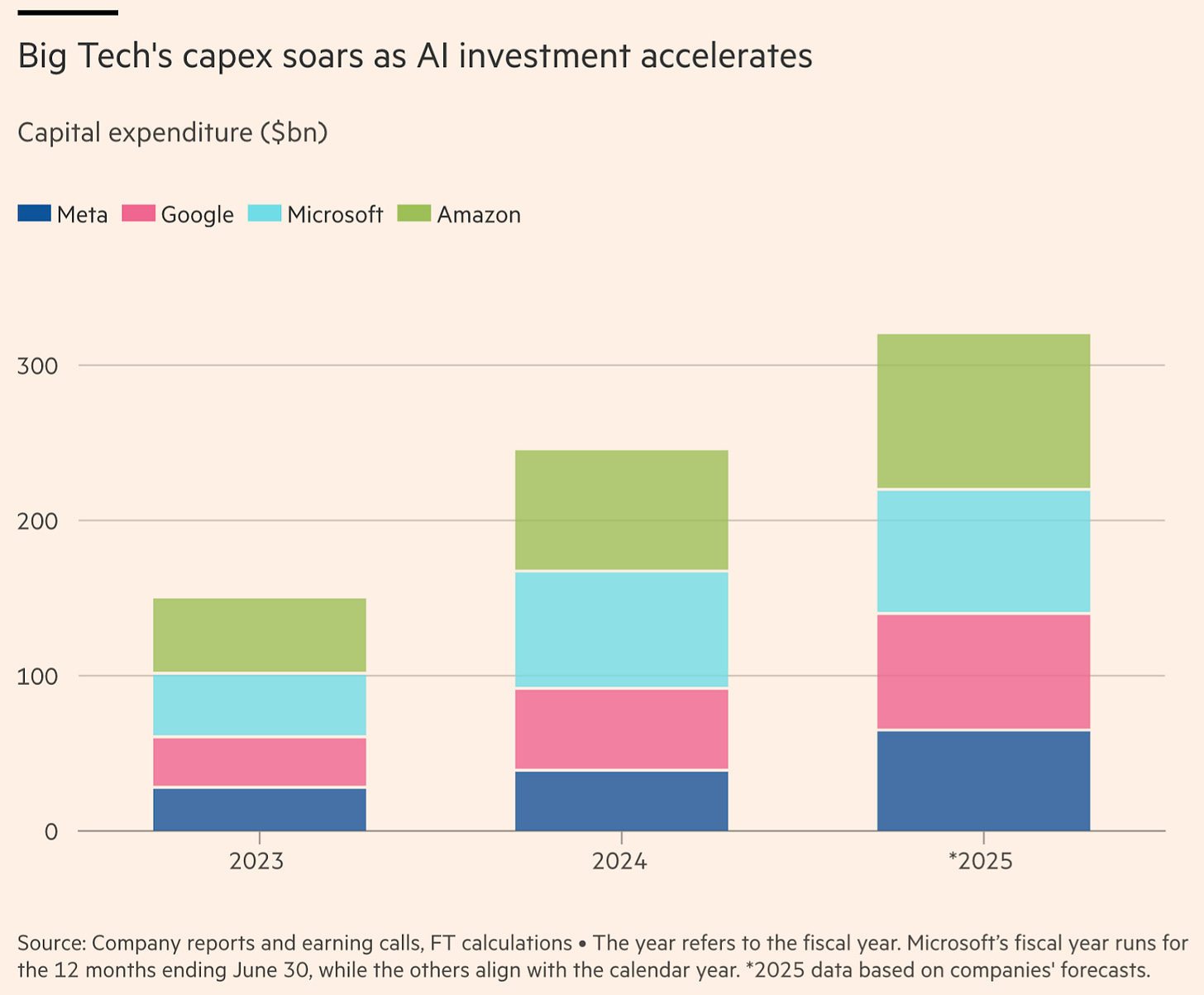
Amazon’s Andy Jassy emphasized AI as a once-in-a-lifetime opportunity and noted current compute constraints:
“The vast majority of that CapEx spend is on AI for AWS. It’s the way AWS business works and the way the cash cycle works— the faster we grow, the more CapEx we spend because we have to procure data center and hardware and chips and networking gear ahead of when we’re able to monetize it… AI represents, for sure, the biggest opportunity since cloud and probably the biggest technology shift and opportunity in business since the Internet.”
"it is true that we could be growing faster, if not for some of the constraints on capacity"
Meta’s Mark Zuckerberg believes that heavy AI infrastructure investment will be a long-term competitive advantage:
“I continue to think that investing very heavily in CapEx and infra is going to be a strategic advantage over time. It’s possible that we’ll learn otherwise at some point, but I just think it’s way too early to call that. And at this point, I would bet that the ability to build out that kind of infrastructure is going to be a major advantage for both the quality of the service and being able to serve the scale that we want to.”
Google’s CFO Ruth Porat projected a $75 billion CapEx spend for 2025:
“As we expand our AI efforts, we expect to increase our investments in capital expenditure for technical infrastructure, primarily for servers followed by data centers and networking. We expect to invest approximately $75 billion in CapEx in 2025.”
While AI efficiency gains may lower some costs, Big Tech is doubling down on infrastructure spending, believing that owning and controlling large-scale AI compute will be a lasting competitive advantage, in the face of already facing capacity constraints early on in the cycle.
Closing Thoughts
In summary, Big Tech’s earnings calls provided a clear picture of how DeepSeek is influencing AI strategy. Five key takeaways emerged:
Training efficiency breakthrough: There is real innovation in what DeepSeek’s accomplish.
Quick diffusion of learnings: AI improvements spread quickly and companies will continuously leapfrog each other, reducing the lifespan of any competitive edge.
More apps and more spending despite lower costs: Lower AI costs will fuel greater adoption, shifting value creation to the application layer
Inference favors strong business models: Companies with sustainable business models will benefit most from AI’s increasing reliance on inference-heavy compute.
Infrastructure as a moat: Big Tech continues massive AI CapEx investments, seeing compute scale as a long-term strategic advantage.
"Inference favors strong business models: Companies with sustainable business models will benefit most from AI’s increasing reliance on inference-heavy compute."
Seems like training heavy compute would have the same takeway no?
"Infrastructure as a moat: Big Tech continues massive AI CapEx investments, seeing compute scale as a long-term strategic advantage."
Infrastructure might be a most right now but I could see that pretty easily being a huge liability if scaling laws stop and enterprises can't find profitable ways to deploy generative AI apps. I think apple's second mover advantage to wait and see this race to AGI is more prudent than herd mentality, granted their apple intelligence product sounds terrible.